Introduction
The data science lifecycle encompasses various stages that data scientists typically go through when working on a project, from understanding the problem statement to delivering a solution. For working professionals, more than a conceptual knowledge, the application of data sciences is more important. A basic knowledge of how the data science lifecycle works in real-life scenarios is essential to gain this practical knowledge. Learning centers in cities where there is enrolment from a substantial number of professionals or students who intent take up data science as a profession offer courses that focus on the application of technologies. Thus, a Data Science Course in Pune , in Bangalore or other tech-oriented cities would include extensive project-based training.
The Data Science Lifecycle
Here’s an overview of the key stages comprising a data science lifecycle:
- Problem Definition: The first step is to clearly define the problem you are trying to solve. This involves understanding the business context, defining project objectives, and identifying key stakeholders.
- Data Collection: Once the problem is defined, gather relevant data from various sources. This may include structured data from databases, unstructured data from text documents or images, and external data from APIs or web scraping.
- Data Cleaning and Preprocessing: Raw data often contains errors, missing values, and inconsistencies. Data cleaning involves identifying and addressing these issues to ensure the quality and reliability of the data. Preprocessing may also involve transforming the data into a suitable format for analysis. Preparing data for analysis is a fundamental step in data analysis and forms an essential topic covered in any Data Science Course.
- Exploratory Data Analysis (EDA): Explore the data to gain insights and understand its characteristics. EDA involves summarising key statistics, visualising distributions, identifying patterns, and uncovering relationships between variables.
- Feature Engineering: Feature engineering involves creating new features or transforming existing ones to improve the performance of machine learning models. This may include scaling, encoding categorical variables, creating interactions, or extracting relevant information from text or images.
- Model Development: Select appropriate machine learning algorithms and train predictive models using the prepared data. This stage involves splitting the data into training and testing sets, tuning model hyperparameters, and evaluating model performance using suitable metrics. There are several levels of model development. An advanced Data Science Course will include lessons on developing complex models while a basic course will cover only the basic concepts of model development. However, developing predictive models is an imperative step in the data science lifecycle.
- Model Evaluation and Validation: Assess the performance of trained models using validation techniques such as cross-validation or holdout validation. Evaluate models based on metrics relevant to the problem domain and consider factors like accuracy, precision, recall, and F1-score.
- Model Deployment: Once a satisfactory model is developed and validated, deploy it into production. This involves integrating the model into existing systems or applications, setting up APIs for inference, and ensuring scalability, reliability, and security.
- Monitoring and Maintenance: Continuously monitor the deployed model’s performance in production to detect drift, anomalies, or degradation in performance. Update the model periodically with new data or retrain it with updated algorithms to maintain its effectiveness over time.
- Documentation and Reporting: Document the entire data science process, including problem formulation, data sources, methodologies, and findings. Prepare reports or presentations to communicate insights, recommendations, and limitations to stakeholders. Most of the engineering and science stream studies are sharply focused on their respective areas and do not cover documentation as part of technical studies. This leaves documentation as a sticky wicket for most technical professionals. This gap in technical studies is addressed in an inclusive Data Scientist Course in Pune and other cities, which would impart documentation skills as well.
- Feedback Loop: Encourage feedback from stakeholders and end-users to iteratively improve the solution. Incorporate feedback into future iterations of the project and adapt to changing requirements or new insights.
Conclusion
By following this structured data science lifecycle, data scientists can effectively navigate through the various stages of a project, from understanding the problem to delivering actionable insights and solutions. While a university course in data science will serve to provide one with the theoretical concepts of data science, for gaining a practical or application-oriented perspective of this technology, attending a bootcamp training or a Data Science Course for professionals is an option worth exploring.
Business Name: ExcelR – Data Science, Data Analyst Course Training
Address: 1st Floor, East Court Phoenix Market City, F-02, Clover Park, Viman Nagar, Pune, Maharashtra 411014
Phone Number: 096997 53213
Email Id: enquiry@excelr.com
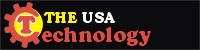